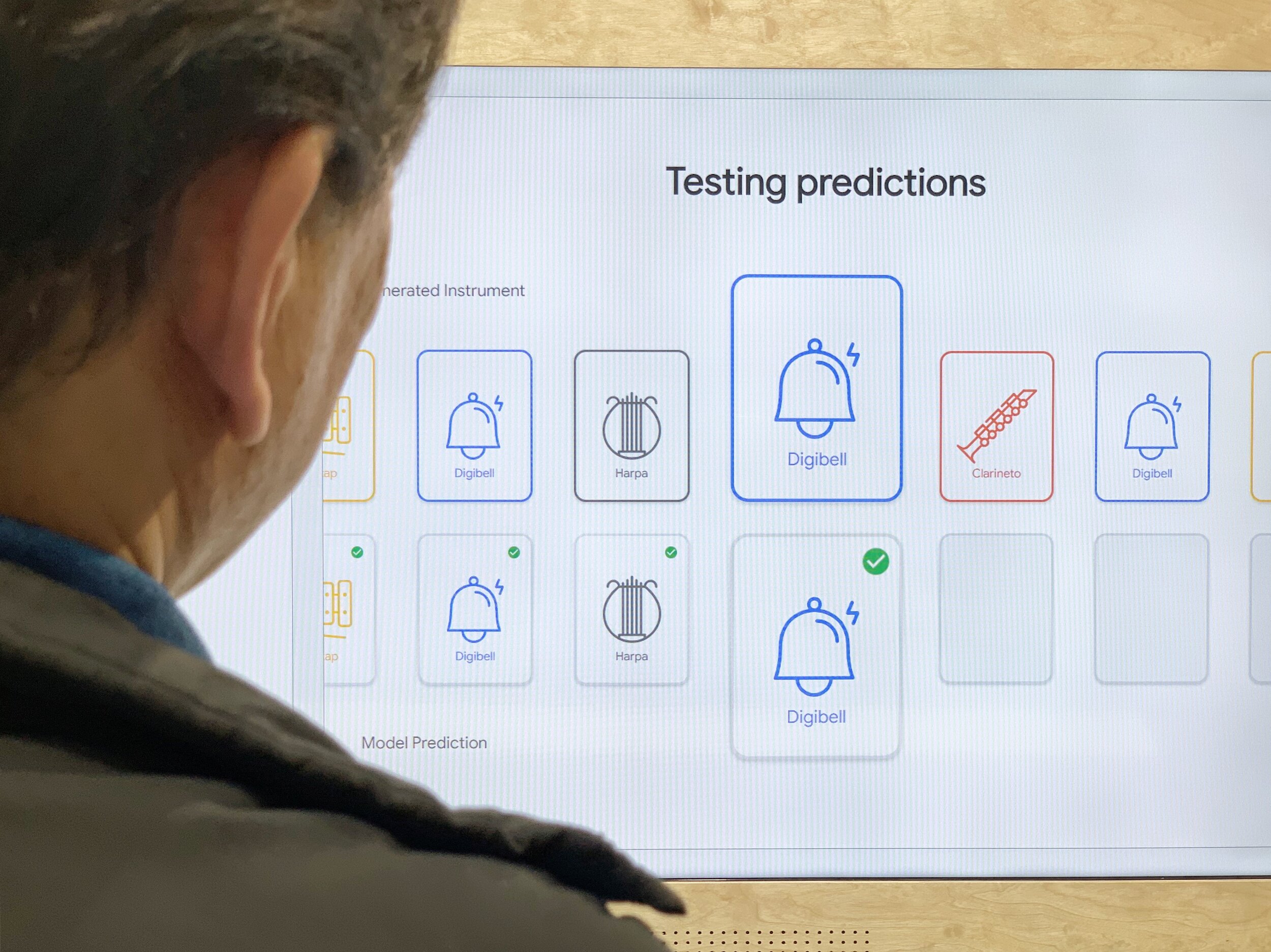
Sounds and Models: A Machine Learning Journey
Google Cloud
Experience Design // Interactive Design
Agency: Sparks

Leveraging the power of Machine Learning can be a challenging concept for (potential) customers to wrap their heads around. In partnership with Google Cloud, our team created an accessible, yet rich experience that shows how easily a machine learning model can be trained and deployed—all through the metaphor of making music. Our work spanned the entire experience: from strategy, defining the user experience, 3D design, visual design, development, and fabrication.
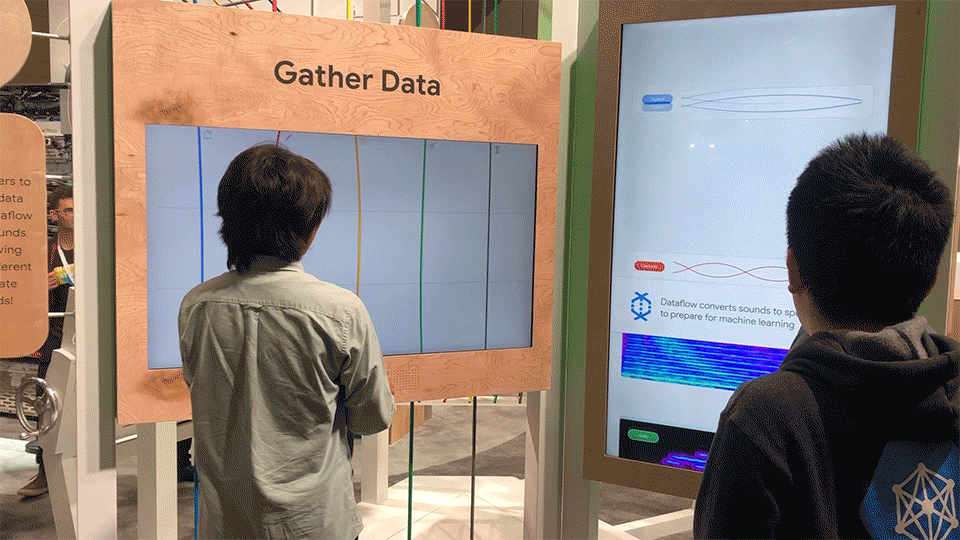
At the first station, attendees gather (create) data by plucking strings on this large-scale experiential instrument. As they create sounds, the sound waves are converted into spectrograms, yielding a visual file that can be easily understood by image recognition tools.

Each string represents a unique instrument, and connects above and below to the surrounding architecture. Depending on where a string is touched, a different note is played.

Different states of the sound conversion station.
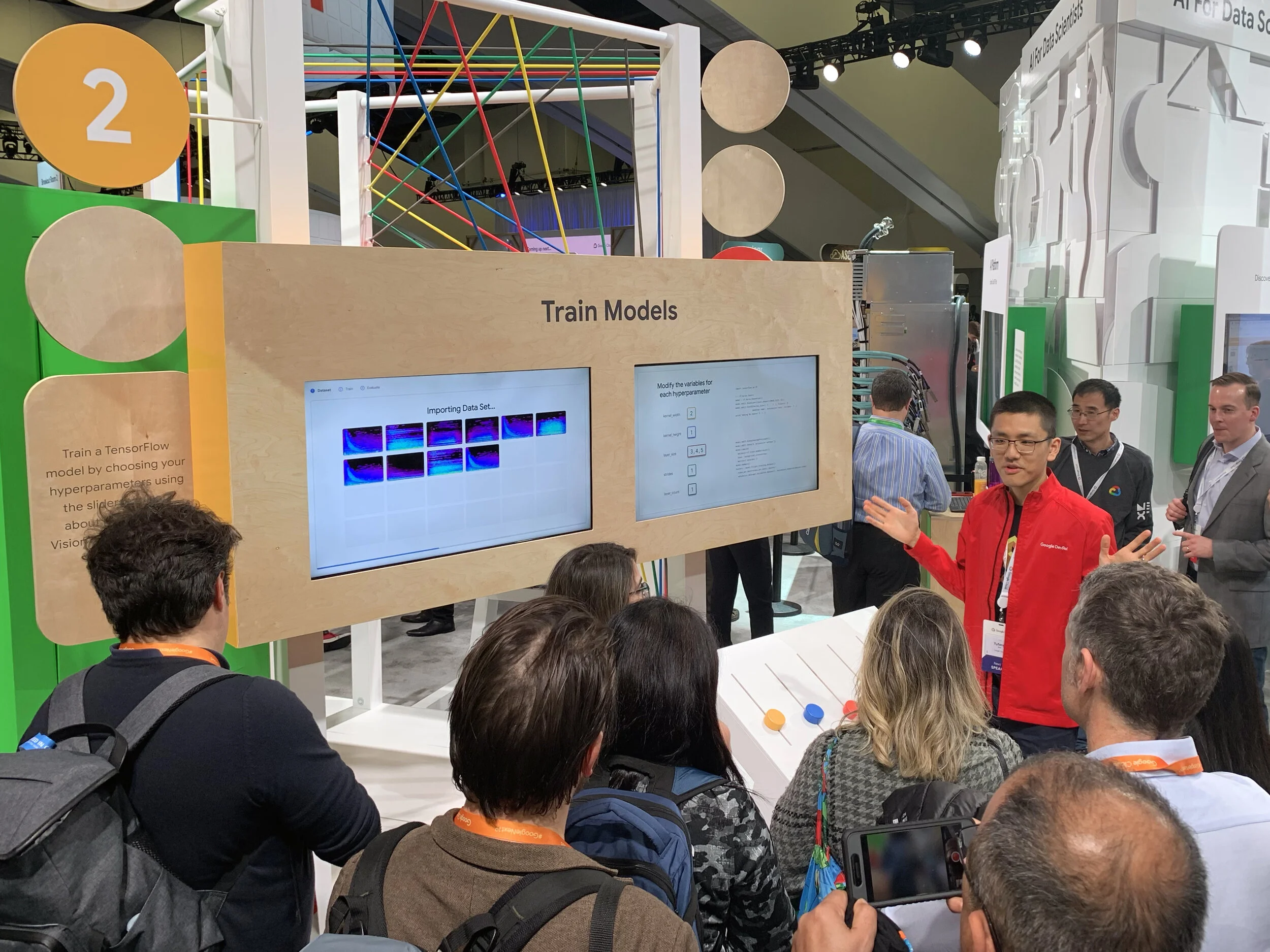
At the second station—leveraging the data they created at the previous station—attendees train a Machine Learning model.
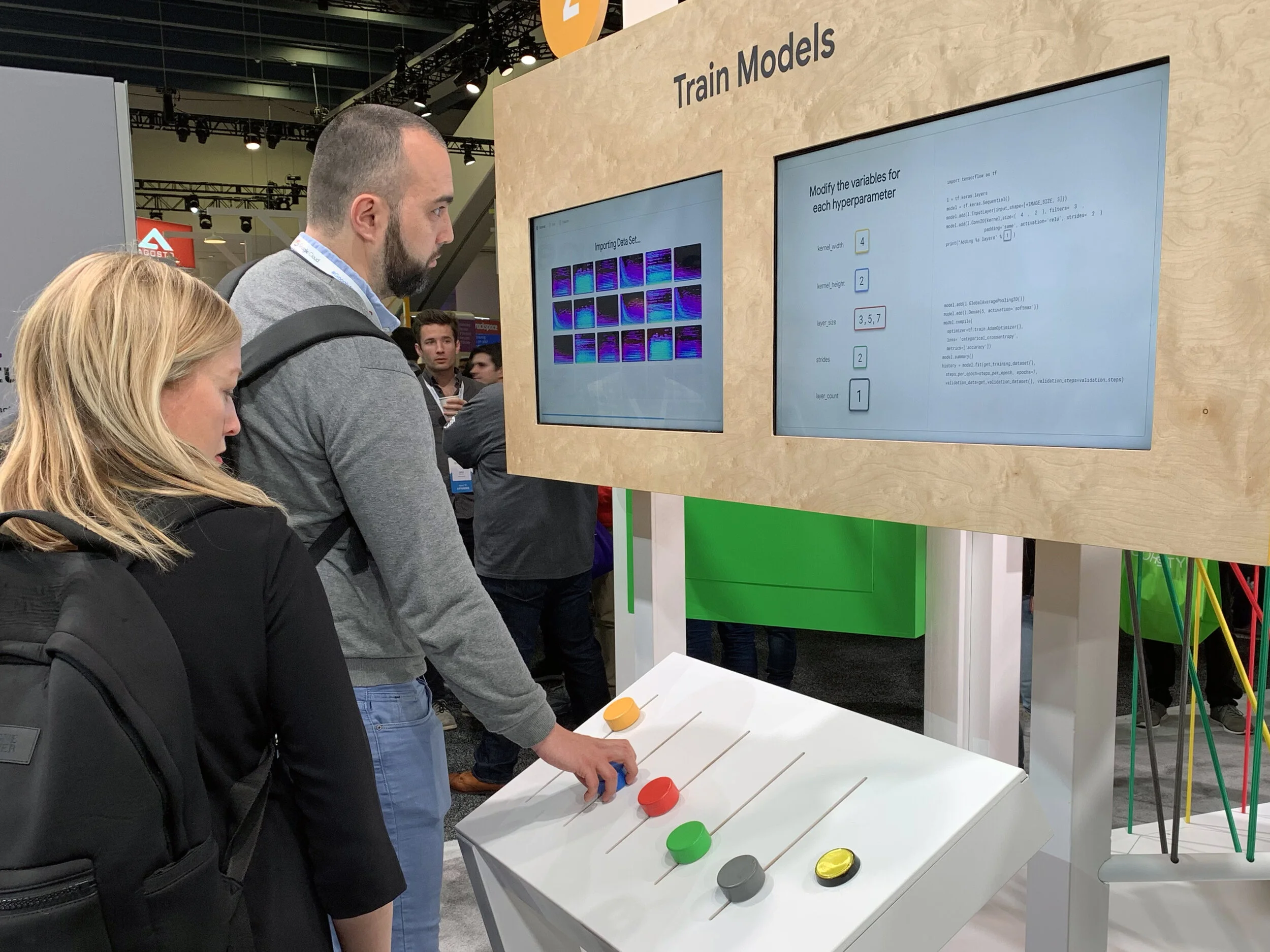
They choose to do this automatically with AutoML, or manually, by adjusting hyper parameters through tactile sliders on a playful mixing board.
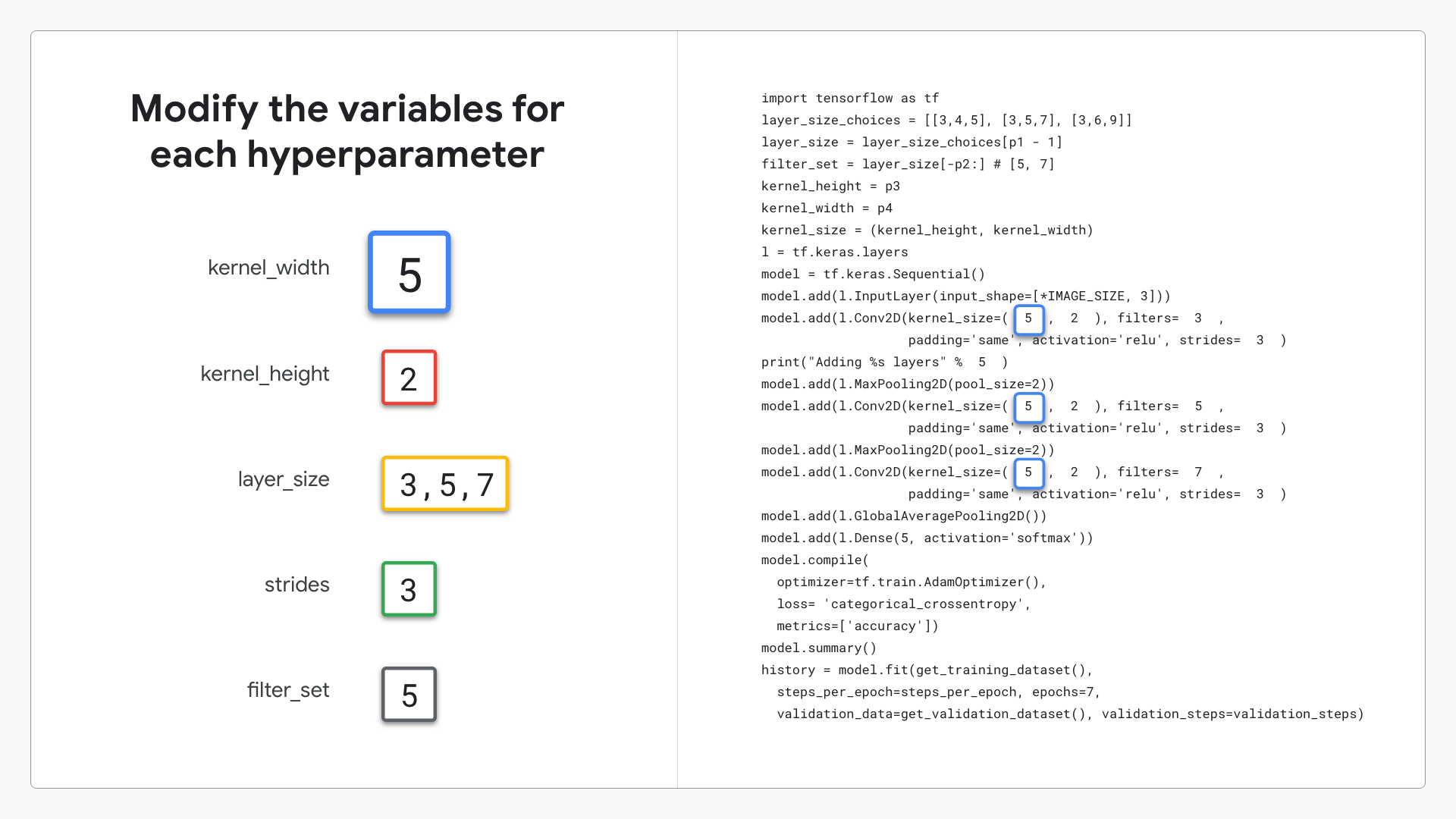
Hyper parameters are adjusted by sliding the dials on the mixing board.
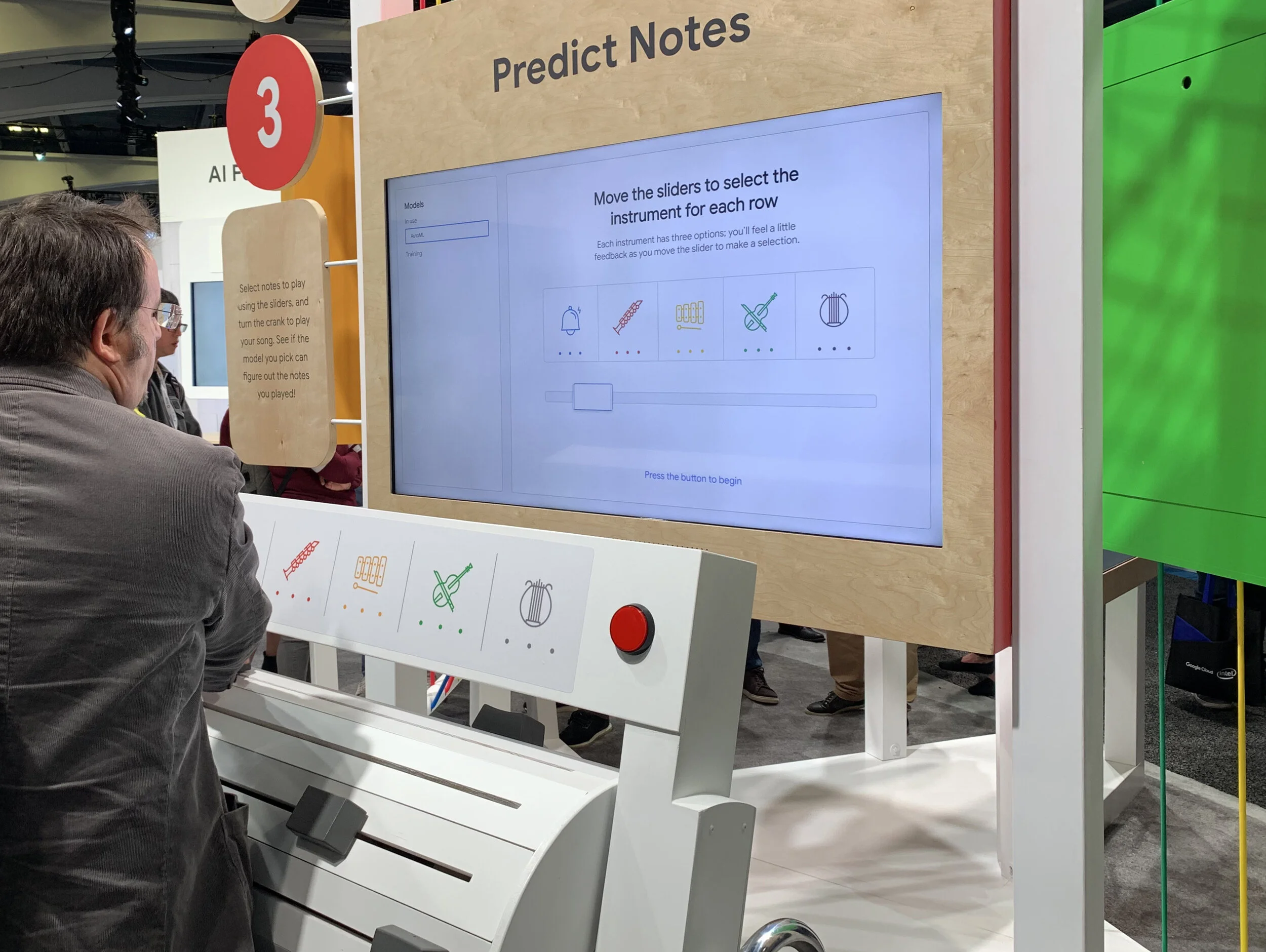
At the third station, attendees test the effectiveness of their model by composing and playing a song on the large-scale music box. Detents on each slider provide tactile feedback for an enhanced note composing experience.

Attendees choose which model to use; the one they created, the AutoML model, or one of many others.
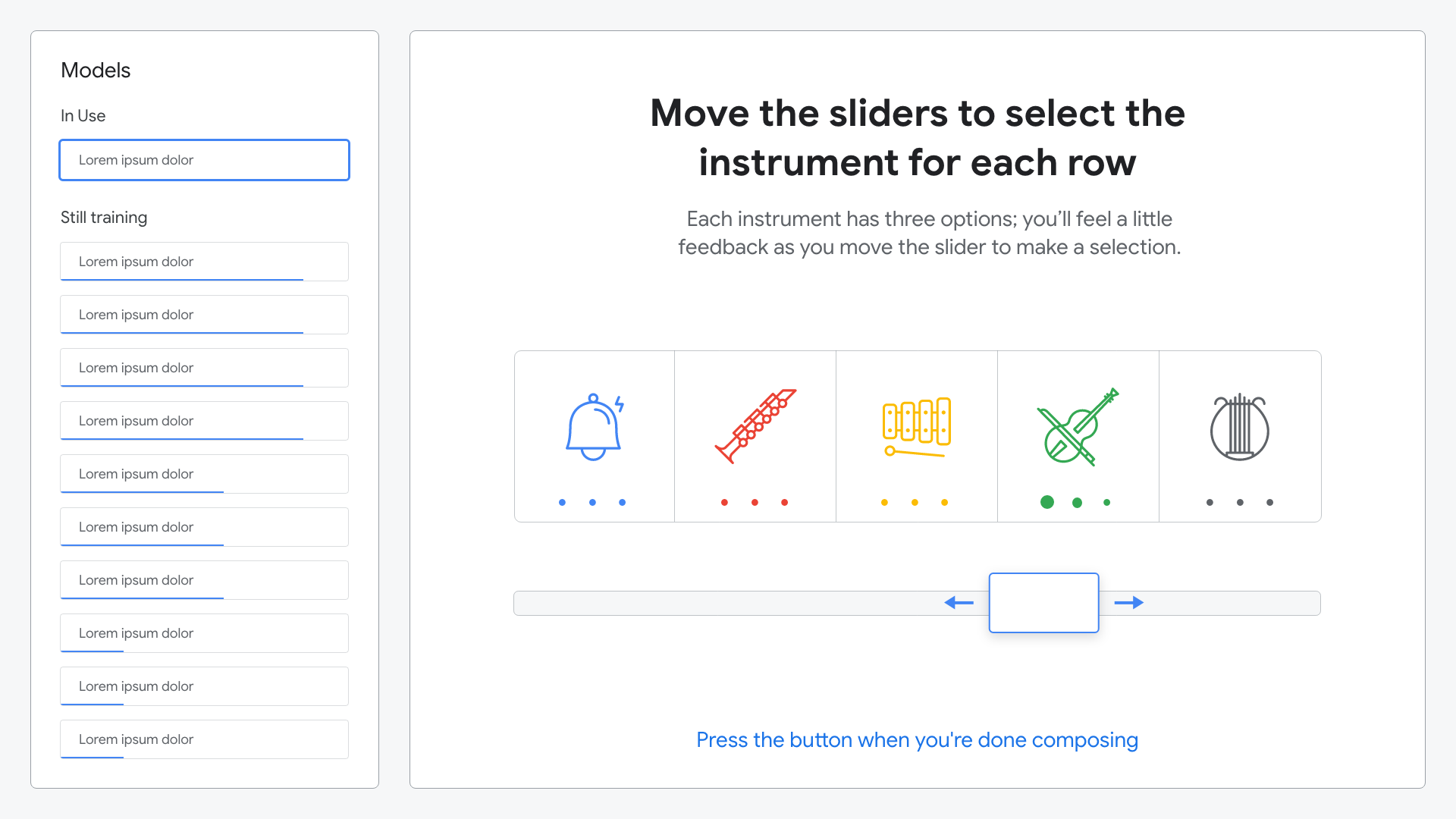
A simple animation guides attendees through through instrument selection.
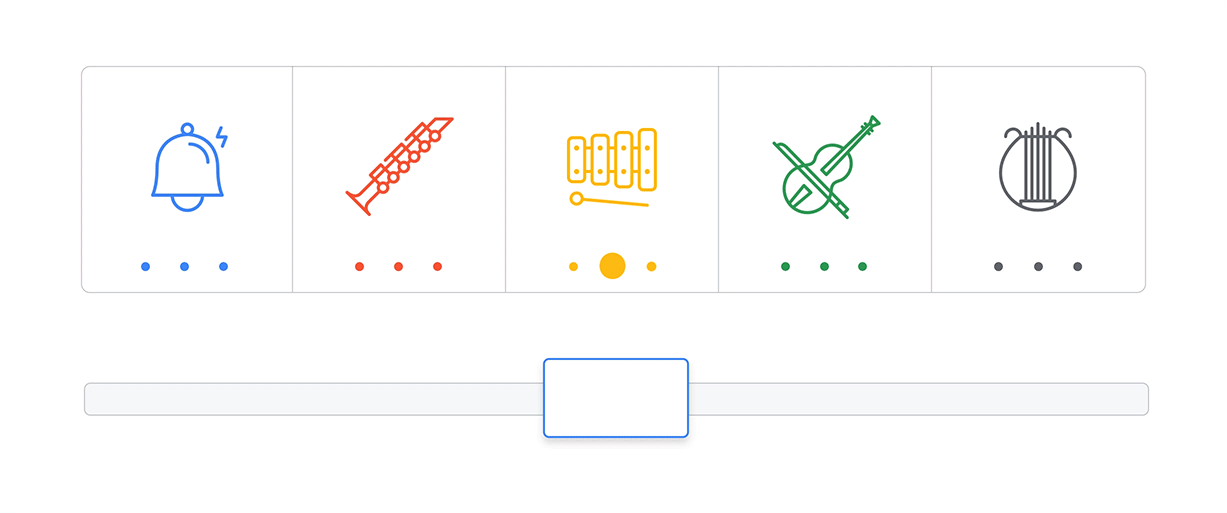
Each dot corresponds to a tactile detent on the physical slider. Depending on where the slider is positioned, a different note from that instrument is played.
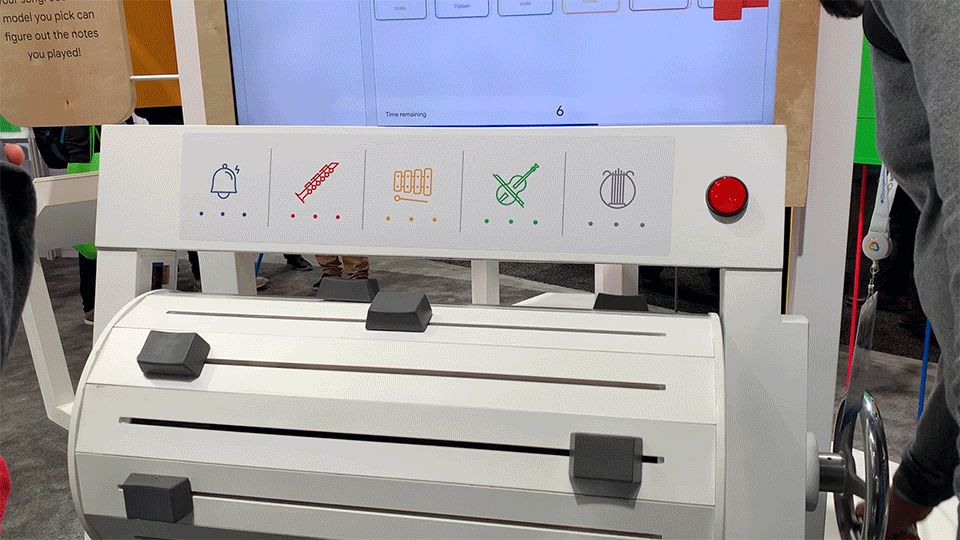
Attendees spin the crank on the large-scale music box to play their notes.
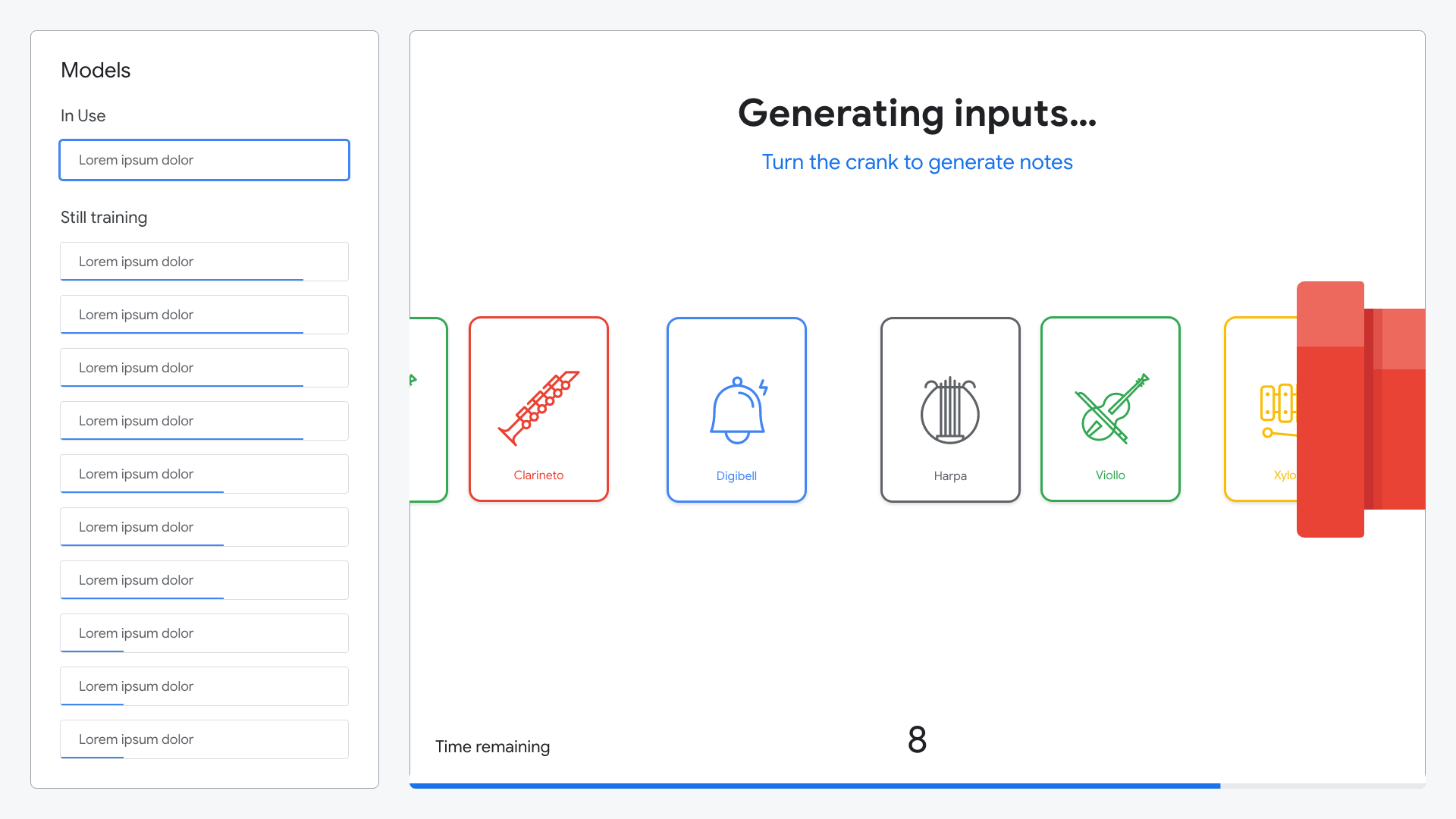
The sound plays audibly and is captured on-screen in real time.
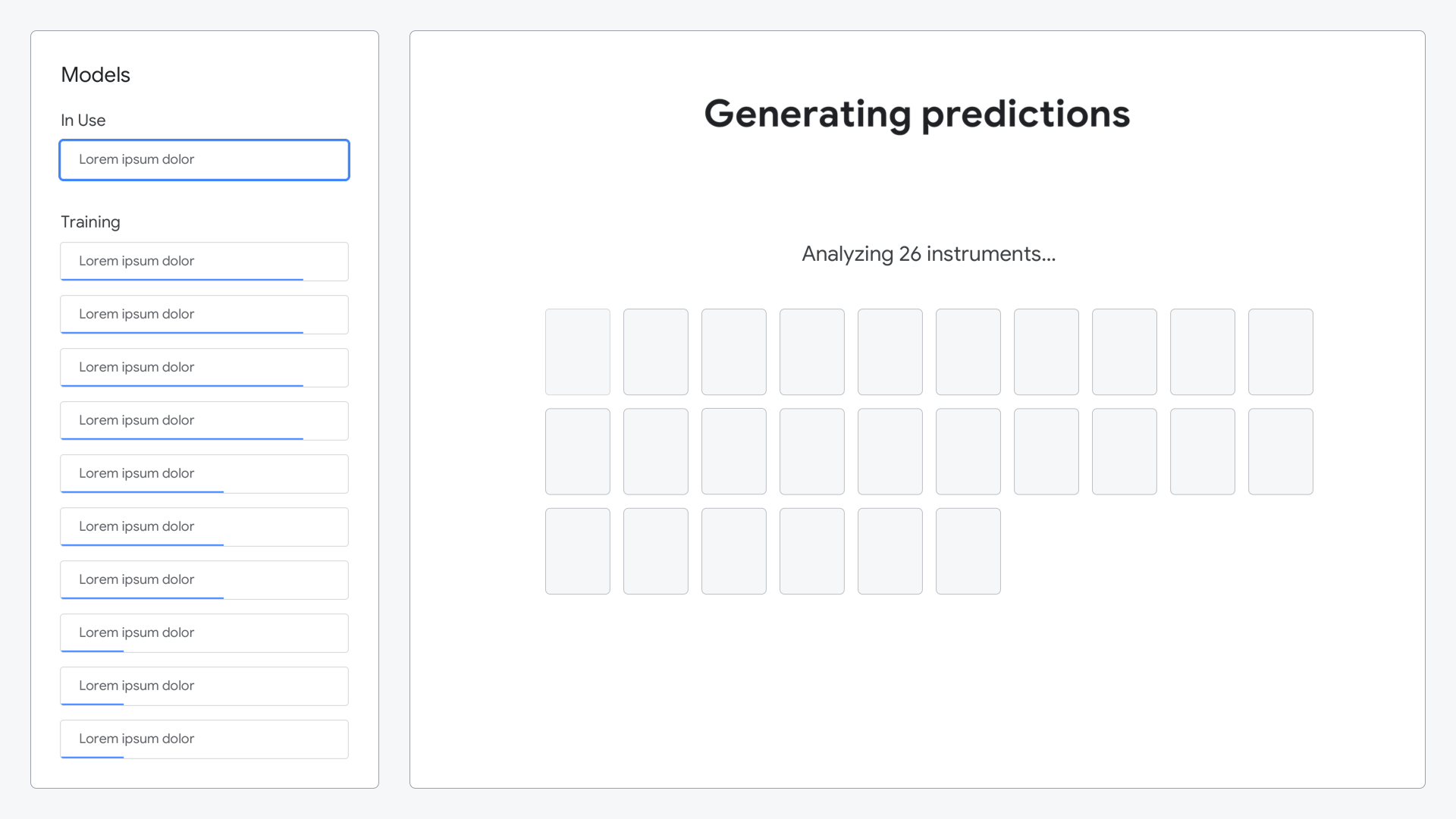
Real-time predictions come back as they’re ready, so a simple animation builds to show this progress.
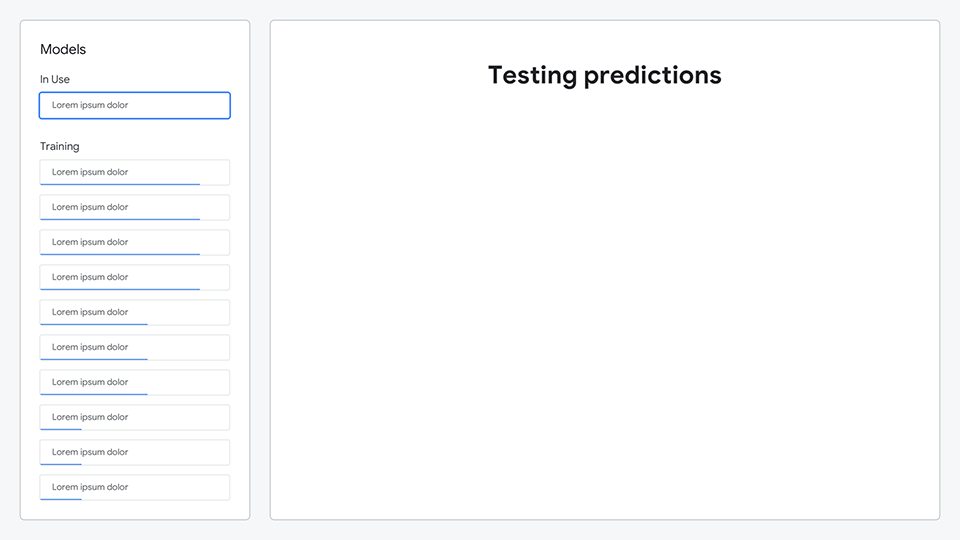
Leveraging the trained model, predictions for each instrument are made and presented against the actual instruments, showing how accurate the model was.
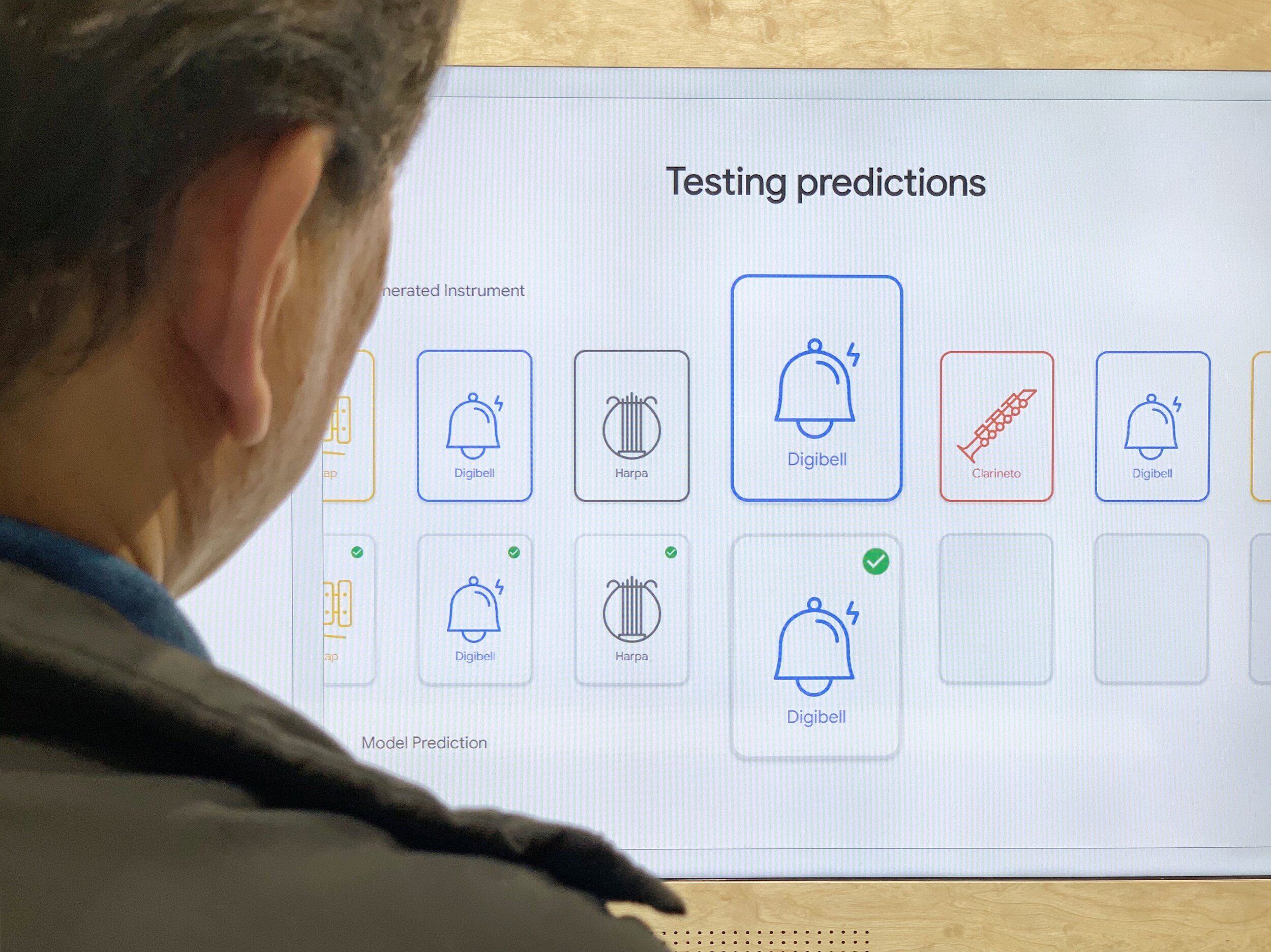
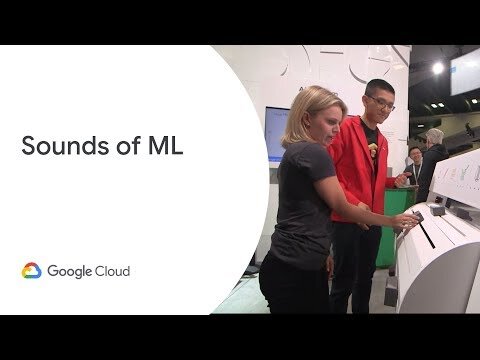
A full demonstration of all three stations.
Sparks Team
SVP, Creative Technology: Jamie Barlow, Creative Director (Interactive): Thomas Ryun, Interactive Designer: Ryan Greenhalgh, Senior Creative Technologist: Marcus Guttenplan, Creative Technologist: Justin Lui, Digital Producer: Jai Sayaka, Creative Director (3D): James Feser, Designer (3D): Mike Roth, Event Manager: Tyler Adamson